Predicting Alcohol Dependence
This work was published in the journal of Human Brain Mapping in October of 2020, and can be found here.
This project was to identify neuroimaging biomarkers of alcohol dependence (AD) from structural magnetic resonance imaging. Data were grouped into a training set used for internal validation including 1,652 participants (692 AD, 24 sites), and a test set used for external validation with 382 participants (146 AD, 3 sites).
This project included an exploratory data analysis, followed by a novel evolutionary search based feature selection procedure, designed to select to highest performing and more generalizable subset of brain measurements.
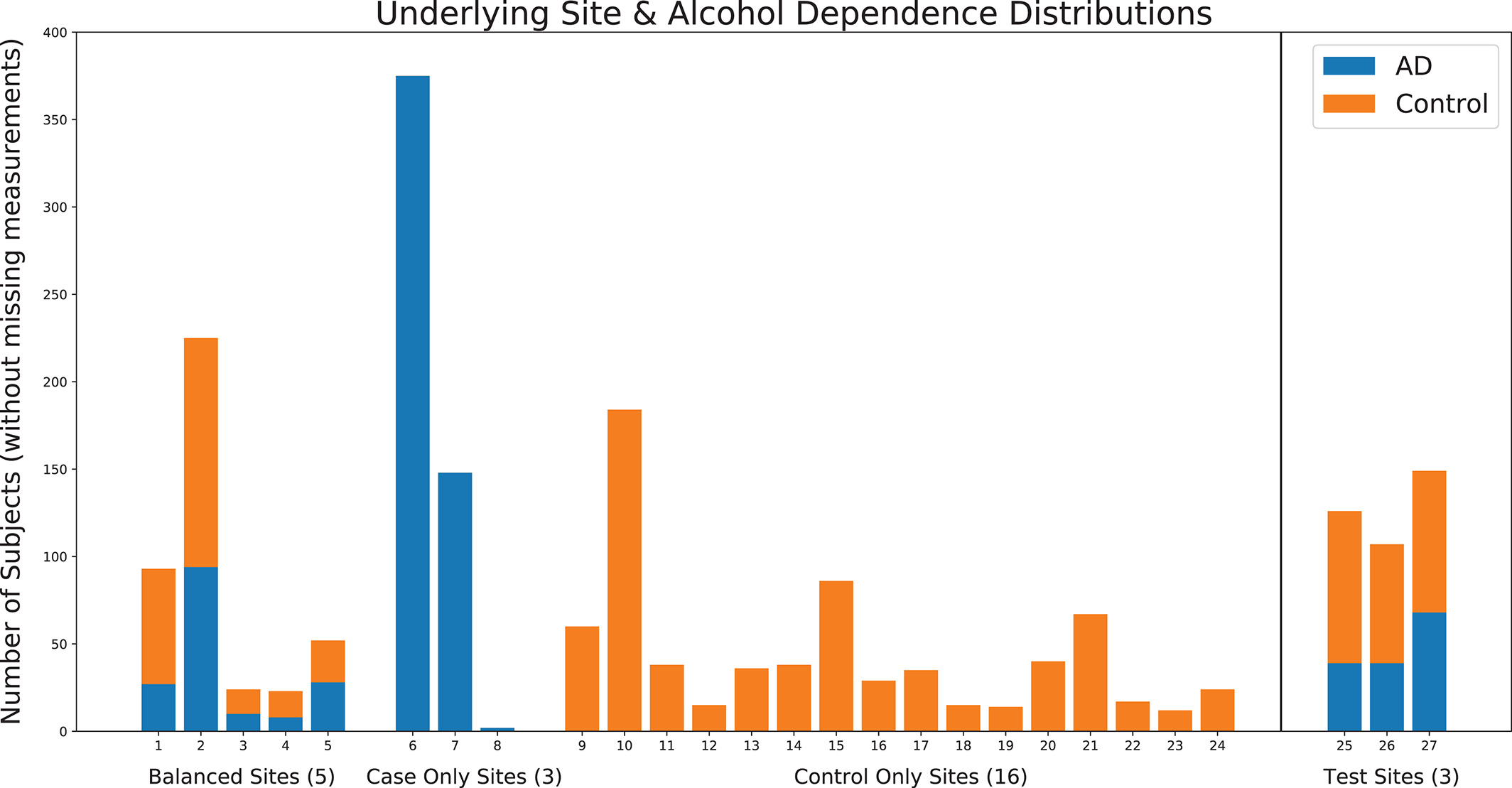
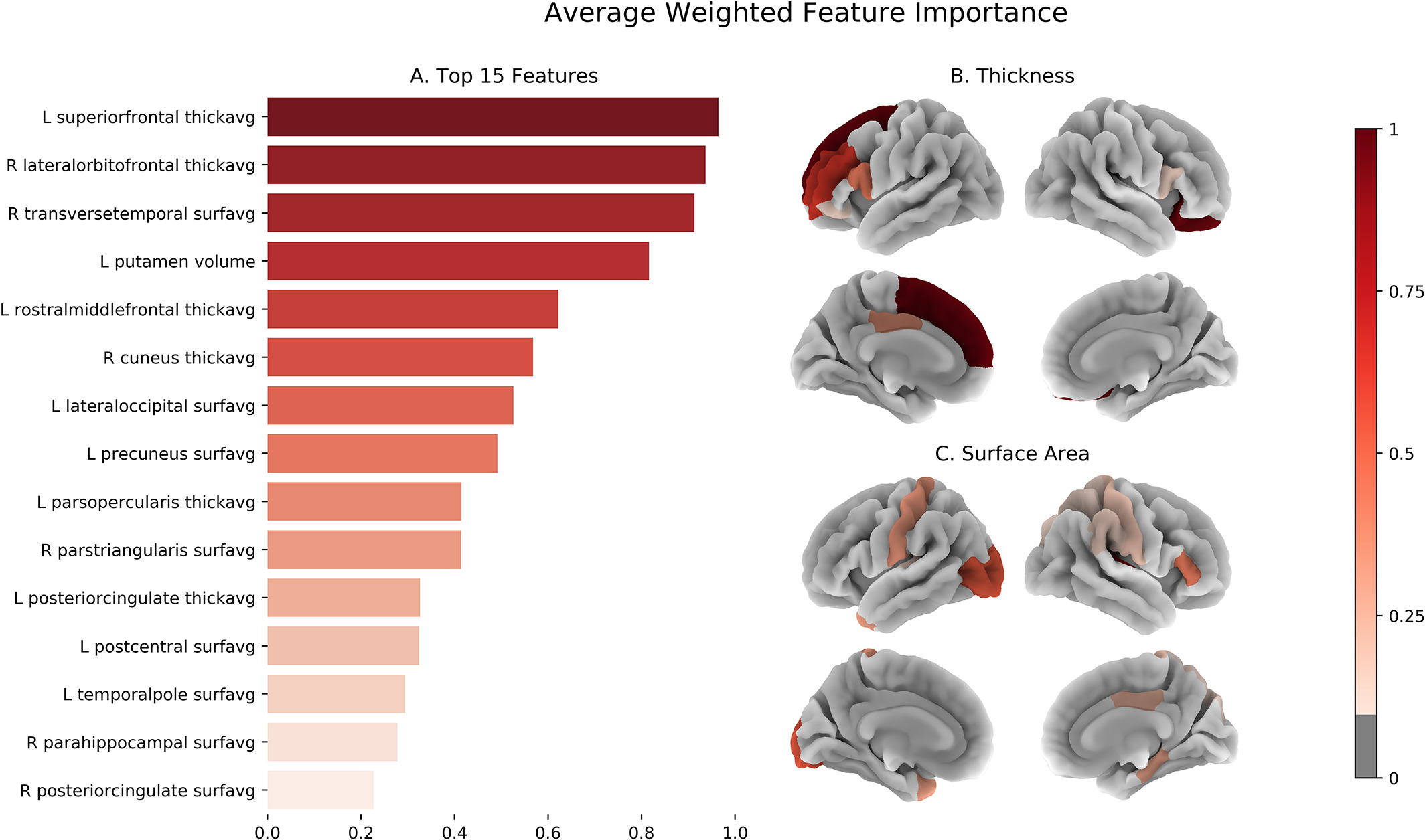